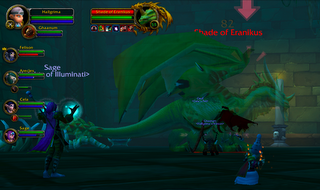
You get a group of folks to agree that, when the dungeon is done or when they leave the party, they will give you a signal: 1, 2, 3, where 1 = great; 2 = okay; and 3 = awful. You capture the chat, as a participant observer, and hopefully capture the video stream. When done, folks give rating. You do a debrief with as many as you can get to show up. You tape their remarks (Second Life would be good for this.)
You go back through your data sets, where one set = one dungeon, BG, or raid (with chat, video, ratings, and hopefully debrief). You sort them by ratings, taking care to put disputed sets (mixed ratings) in their own pile. You go through each pile of datasets trying to identify common themes in that set. Then you look across sets at what differentiates the good, the bad, and the mediocre...and the mixed bag. What do the good ones have in common? How do they differ from the others?
Maybe it will be leadership. Maybe it will be communication. Maybe it will experience (expertise in group). etc etc.
At write up, you make the case for dungeons or BGs or instances as cases of a particular kind of collaborative work. If this kind of collaborative work occurs in the real world, at work or at school or in-between, you can make discuss what you've found out about what makes more and less successful task or project collaborations.
You could do something similar with guilds... You have to have insider ratings to use to differentiate the variations based upon criteria identified by the users, e.g.,BG twink guilds serve different needs than endgame raiding guilds. The you look in the clearly demarcated grouping for what is common within the group, and how the grouping is differentiated from others.
These two studies should also give you a predictive power that you can check out. Then, of course, you'll want to move it to a real world setting and see if the principles hold true.
No comments:
Post a Comment